NEXT STORY
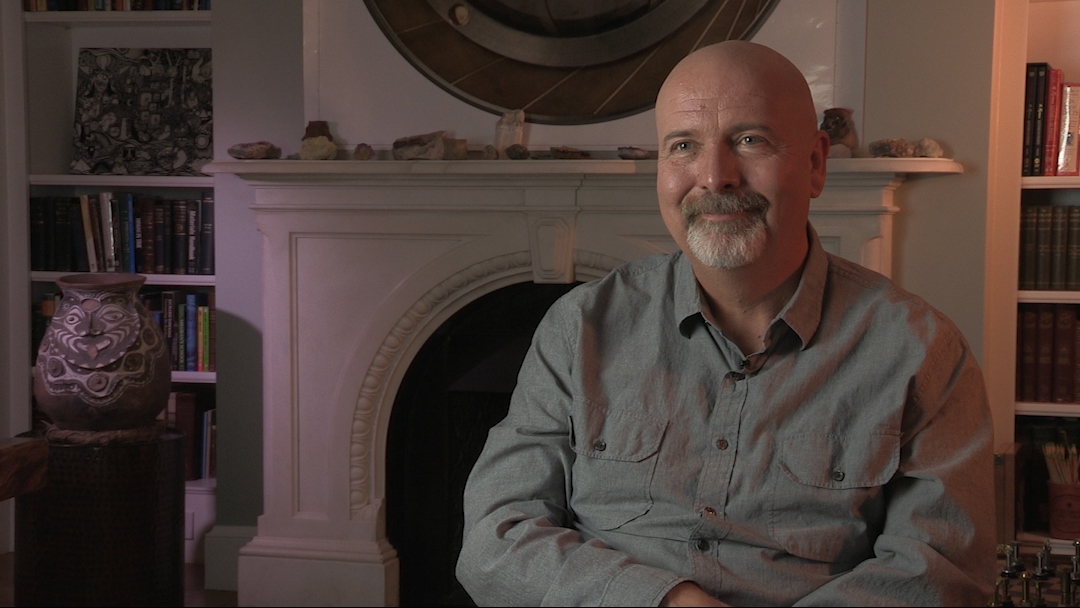
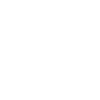
My interest in proteomics
RELATED STORIES
NEXT STORY
My interest in proteomics
RELATED STORIES
![]() |
Views | Duration | |
---|---|---|---|
181. AI and why I built the Connection Machine | 96 | 03:24 | |
182. The complexity of human intelligence | 98 | 05:03 | |
183. Recreating evolution inside a computer | 1 | 78 | 03:38 |
184. Nature – the great engineer | 1 | 89 | 02:44 |
185. Morphogenesis as an adaptive process | 74 | 03:01 | |
186. The two-dimensional landscape of evolution | 73 | 03:51 | |
187. Evolving an intelligence with the use of computers | 68 | 00:59 | |
188. Programming an intelligence for solving complex problems | 65 | 03:36 | |
189. How to create an intelligence | 66 | 05:11 | |
190. My interest in proteomics | 81 | 02:50 |
What we'd really like, if we had another kind of intelligence, I think, would be an intelligence that could look at a very complicated system and suggest how we might change its inputs a little bit in order to keep it operating in the safe zone. So let's take that as a problem. How would we create that intelligence? So I would start out with a very simple version of that problem, which is imagine you have a little box. Technically it would be a finite state machine. And it has some switches and knobs on it. And it has some lights on it. And your job is to control the switches and knobs so that the light... some lights stay on and some lights stay off. Now I can start... I can make that box as simple as I want. I can have one light on it and one switch. It would be really simple. And so it would be easy to make a machine that learned when the switch is in this position, the light is on, and when the switch is in that position, the light is off. And you could imagine that the box might even have some internal states, so maybe I have to turn on the switch twice to get the light to turn on. And the machine could learn that, it could build a model of that. And I could increase the number of switches and lights and knobs and the complexity of the algorithm inside the box, gradually, and have the machine get better and better at learning how to control it. And at some point, I'm imagining that it's doing this and it's accumulating the techniques that it used before, and it's using those as building blocks for the next level of evolution of the machine. And so I could get... maybe the next level of complexity is like playing a video game, which is basically you see some lights on the screen and you have some controls and the state is in the game and you learn to control the switches in order to make the score go up. In that case, you know, you're... so you have a goal state which is a high score, and you have some controls, and you learn by playing with it what to do to get the high score.
And in fact, neural networks have done that, so we know machines can kind of learn to do that, but I can keep on making it more and more complicated, till eventually you have something as complicated as, say, a human body that you're putting medicines into or something like that. Of course, you start with simulations. But I think that's a nice kind of framework that you can make arbitrarily complicated. So that's the framework I think I would use to evolve these machines. I'd start them out on simple machines, they'd get good at it, and then I'd build up a more and more complicated situation. And I might even do a kind of co-evolution thing, where the machines also evolved and they tried to evolve to be inscrutable and picky ways of being controlled and so on, so that... like the sorting programs and the...
And so I think that program, if you didn't just evolve a set of instructions, but what you evolved is something which, by an adaptive process, produced a program. And maybe it's by an automatic debugging and, you know, made a more sensible program from the genes. And then that program learned from interaction with the environment. You get those multiple levels of adaptation. Then I think you would get that kind of hierarchical evolution that I was talking about before. And I think you might be able to evolve a kind of intelligence that would surpass human intelligence and actually help us with some of these big problems that we have that we don't know how to solve. So there's a program of research that I would really love to figure out how to do somehow.
[Q] So when do you start?
Well, I'm thinking of the best way to do it. I mean, obviously, you need to get some great students or colleagues working on it. You need a lot of computing resources. I think Boston would actually be a wonderful place to do it. You need some people that... you know, it's a big programming project in creating the environment and so on. I don't think it's... it's not obvious to me how to build the organisation. I'm at a point... I've built organisations before, but I realise that's not really what I want to do. I'm not like an institute builder, so I kind of need to figure out how to do this and get somebody else to build the organisation and have, hopefully, me work on the actual technology. So I haven't figured that out yet. But that's what I'd really like to do.
W Daniel Hillis (b. 1956) is an American inventor, scientist, author and engineer. While doing his doctoral work at MIT under artificial intelligence pioneer, Marvin Minsky, he invented the concept of parallel computers, that is now the basis for most supercomputers. He also co-founded the famous parallel computing company, Thinking Machines, in 1983 which marked a new era in computing. In 1996, Hillis left MIT for California, where he spent time leading Disney’s Imagineers. He developed new technologies and business strategies for Disney's theme parks, television, motion pictures, Internet and consumer product businesses. More recently, Hillis co-founded an engineering and design company, Applied Minds, and several start-ups, among them Applied Proteomics in San Diego, MetaWeb Technologies (acquired by Google) in San Francisco, and his current passion, Applied Invention in Cambridge, MA, which 'partners with clients to create innovative products and services'. He holds over 100 US patents, covering parallel computers, disk arrays, forgery prevention methods, and various electronic and mechanical devices (including a 10,000-year mechanical clock), and has recently moved into working on problems in medicine. In recognition of his work Hillis has won many awards, including the Dan David Prize.
Title: How to create an intelligence
Listeners: Christopher Sykes George Dyson
Christopher Sykes is an independent documentary producer who has made a number of films about science and scientists for BBC TV, Channel Four, and PBS.
Tags: intelligence, neural networks, adaptation, evolution
Duration: 5 minutes, 11 seconds
Date story recorded: October 2016
Date story went live: 05 July 2017